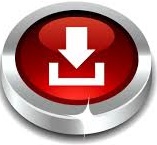

Chest X-ray image data cannot be shared publicly because of the private health information policies of participating institutions. This is an open access article distributed under the terms of the Creative Commons Attribution License, which permits unrestricted use, distribution, and reproduction in any medium, provided the original author and source are credited.ĭata Availability: All relevant data except for chest X-ray images are within the paper and its Supporting Information files. Received: JanuAccepted: Published: June 7, 2021Ĭopyright: © 2021 Hwang et al. PLoS ONE 16(6):Įditor: Jeonghwan Gwak, Korea National University of Transportation, REPUBLIC OF KOREA (2021) COVID-19 pneumonia on chest X-rays: Performance of a deep learning-based computer-aided detection system. In conclusion, radiologist-level performance of the CAD in identifying COVID-19 and associated pneumonia on CXR and enhanced performance of non-radiologist physicians with the CAD assistance suggest that the CAD can support physicians in interpreting CXRs and helping image-based triage of COVID-19 patients in resource-constrained environment.Ĭitation: Hwang EJ, Kim KB, Kim JY, Lim J-K, Nam JG, Choi H, et al. In addition, inter-reader agreement among physicians was also improved in the CAD-assisted interpretation (Fleiss’ kappa coefficient, 0.209 to 0.322). Non-radiologist physicians showed significantly improved performance when assisted with the CAD (AUCs, 0.584 to 0.664 and 0.650 to 0.738). The performance of CAD (AUCs, 0.714 and 0.790 against RT-PCR and chest CT, respectively hereinafter) were similar with those of thoracic radiologists (AUCs, 0.701 and 0.784), and higher than those of non-radiologist physicians (AUCs, 0.584 and 0.650). Afterward, they re-interpreted the CXRs with corresponding CAD results.

For comparison, 5 thoracic radiologists and 5 non-radiologist physicians independently interpreted the CXRs. Performance of the CAD was evaluated using area under the receiver operating characteristic curves (AUCs), with reference standards of the RT-PCR results and the presence of findings of pneumonia on chest CTs obtained within 24 hours from the CXR. In this study, CXRs of patients with and without COVID-19 confirmed by reverse transcriptase polymerase chain reaction (RT-PCR) were retrospectively collected from four and one institution, respectively, and a commercialized, regulatory-approved CAD that can identify various abnormalities including pneumonia was used to analyze each CXR. However, the performance of existing CAD for identifying COVID-19 and associated pneumonia on CXRs has been scarcely investigated. In order to account for any grading errors, the evaluation set was also checked by a third expert.Chest X-rays (CXRs) can help triage for Coronavirus disease (COVID-19) patients in resource-constrained environments, and a computer-aided detection system (CAD) that can identify pneumonia on CXR may help the triage of patients in those environment where expert radiologists are not available. The diagnoses for the images were then graded by two expert physicians before being cleared for training the AI system. All chest X-ray imaging was performed as part of patients’ routine clinical care.įor the analysis of chest x-ray images, all chest radiographs were initially screened for quality control by removing all low quality or unreadable scans. There are 5,856 X-Ray images (JPEG) in 2 categories (Pneumonia/Normal).Ĭhest X-ray images (anterior-posterior) were selected from retrospective cohorts of pediatric patients of one to five years old from Guangzhou Women and Children’s Medical Center, Guangzhou. The dataset is organized into 2 folders (train, test) and contains subfolders for each image category (Pneumonia/Normal). Pneumonia Detection on Chest X-Rays with Deep Learning Data description
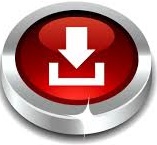